To control access to resources in the Amazon Web Services (AWS) Cloud, customers can author AWS Identity and Access Management (IAM) policies. The IAM policy language is expressive, allowing you to create fine-grained policies that control who can perform what actions on which resources. This control can be used to enforce the principle of least privilege, granting only the permissions required to perform a task.
But how can you verify that your IAM policies meet your security requirements? At AWS’s 2023 re:Invent conference, we announced the launch of IAM Access Analyzer custom policy checks, which help you benchmark policies against your security standards. Custom policy checks abstract away the task of converting policy statements into mathematical formulas, so customers can enjoy the benefits of automated reasoning without expertise in formal logic.
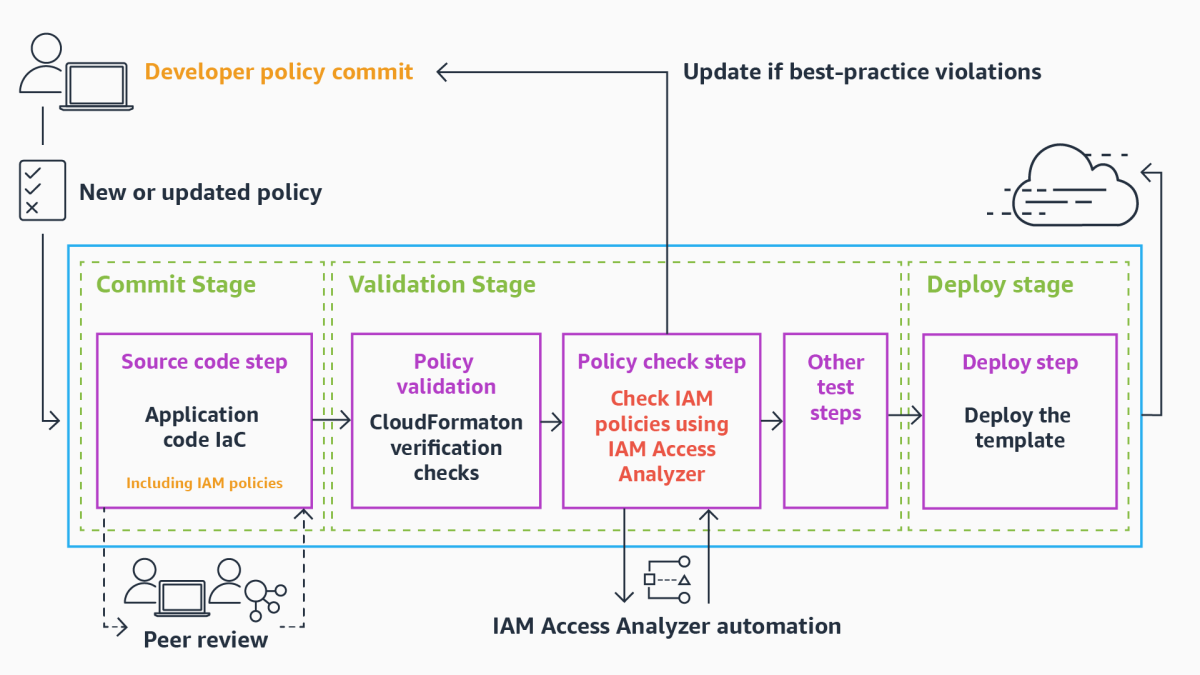
The IAM Access Analyzer API CheckNoNewAccess ensures that you do not inadvertently add permissions to a policy when you update it. With the CheckAccessNotGranted API, you can specify critical permissions that developers should not grant in their IAM policies.
We built custom policy checks on an internal AWS service called Zelkova, which uses automated reasoning to analyze IAM policies. Previously, we used Zelkova to build preventative and detective managed controls, such as Amazon S3 Block Public Access and IAM Access Analyzer public and cross-account findings. Now, with the release of custom policy checks, you can set a security standard and prevent policies that do not meet this standard from being deployed.
How does Zelkova work?
Zelkova models the semantics of the IAM policy language by translating policies into precise mathematical expressions. It then uses automated engines called satisfiability modulo theories (SMT) solvers to check properties of the policies. Satisfiability (SAT) solvers check if it is possible to assign true or false values to Boolean variables to satisfy a set of constraints; SMT is a generalization of SAT to include strings, integers, real numbers, or functions. The benefit of using SMT to analyze policies is that it is comprehensive. Unlike tools that simulate or evaluate a policy for a given request or a small set of requests, Zelkova can check properties of a policy for all possible requests.
Consider the following Amazon S3 bucket policy:
{ "Version": "2012-10-17", "Statement": [ { "Effect": "Allow", "Principal": "*", "Action": ["s3:PutObject"], "Resource": "arn:aws:s3:::DOC-EXAMPLE-BUCKET" } ] }
Zelkova translates this policy into the following formula:
(Action = “s3:PutObject”) ∧ (Resource = “arn:aws:s3:::DOC-EXAMPLE-BUCKET”)
In this formula, "∧" is the mathematical symbol for “and”. Action and Resource are variables that represent values from any possible request. The formula is true only when a request is allowed by the policy. This precise mathematical representation of a policy is useful because it allows us to answer questions about the policy exhaustively. For example, we can ask if the policy allows public access, and we receive the answer that it does.
For simple policies such as the preceding policy, we could perform manual reviews to determine whether they allow public access: the "Principal": "*" in the policy’s statement means that anyone (the public) is allowed access. But manual review can be error prone and is not scalable.
Alternatively, we could write simple syntactic checks for patterns such as "Principal": "*". However, these syntactic checks can miss the subtleties of policies and the interactions between different parts of a policy. Consider the following modification of the preceding policy, which adds a Deny statement with "NotPrincipal": "123456789012"; the policy still has the pattern "Principal": "*", but it no longer allows public access:
{ "Version": "2012-10-17", "Statement": [ { "Effect": "Allow", "Principal": "*", "Action": ["s3:PutObject"], "Resource": "arn:aws:s3:::DOC-EXAMPLE-BUCKET" }, { "Effect": "Deny", "NotPrincipal": "123456789012", "Action": "*", "Resource": "*" } ] }
With the mathematical representation of policy semantics in Zelkova, we can answer questions about access privileges precisely.
Answering questions with Zelkova
As an example, let’s consider a relatively simple question. With IAM policies, you can grant cross-account access to resources you want to share. For sensitive resources, you’d like to check that cross-account access is not possible.
Suppose we wanted to check whether the preceding policies allow anyone outside my account, 123456789012, to access my S3 bucket. Just as we translated the policy into a mathematical formula, we can translate the question we want to ask (or property we want to check) into a mathematical formula. To check whether all allowed accesses are from my account, we can translate the property to the following formula:
(Principal = 123456789012)
To show that the property holds true for the policy, we can now try to prove that only requests with (Principal = 123456789012) are allowed by the policy. A common trick used in mathematics is to flip the question around. Instead of trying to prove that the property holds, we can prove that it does not hold by finding requests that do not satisfy it — in other words, requests that satisfy (Principal ≠ 123456789012). To find such a counterexample, we look for assignments to the variables Principal, Action, and Resource such that the following is true:
(Action = “s3:PutObject”) ∧ (Resource = “arn:aws:s3:::DOC-EXAMPLE-BUCKET”) ∧ (Principal ≠ 123456789012)
Zelkova translates the policy and property into the preceding mathematical formula, and it efficiently searches for counterexamples using SMT solvers. For the preceding formula, the SMT solver can produce a counterexample showing that such access is indeed allowed by the policy (for example, with Principal = 111122223333).
For the previously modified policy with the Deny statement, the SMT solver can also prove that no solution is possible for the resulting formula and that no access is allowed for the policy from outside my account, 123456789012:
(Action = “s3:PutObject”) ∧ (Resource = “arn:aws:s3:::DOC-EXAMPLE-BUCKET”) ∧ (Principal = 123456789012) ∧ (Principal ≠ 123456789012)
The Deny statement in the policy with "NotPrincipal": "123456789012" is translated to the constraint (Principal = 123456789012). By inspecting the preceding formula, we can see that it can’t be satisfied: the constraints on Principal from the policy and from the property are contradictory. An SMT solver can prove this and more complicated formulas by exhaustively ruling out solutions.
Custom policy checks
To democratize access to Zelkova, we needed to abstract the construction of mathematical formulas behind a more accessible interface. To that end, we launched IAM Access Analyzer custom policy checks with two predefined checks: CheckNoNewAccess and CheckAccessNotGranted.
With CheckNoNewAccess, you can confirm that you do not accidentally add permissions to a policy when updating it. Developers often start with more-permissive policies and refine them over time toward least privilege. With CheckNoNewAccess, you can now compare two versions of a policy to confirm that the new version is not more permissive than the old version.
Suppose a developer updates the first example policy in this post to disallow cross-account access but at the same time also adds a new action:
{ "Version": "2012-10-17", "Statement": [ { "Effect": "Allow", "Principal": "123456789012", "Action": [ "s3:PutObject", "s3:DeleteBucket" ], "Resource": "arn:aws:s3:::DOC-EXAMPLE-BUCKET" } ] }
CheckNoNewAccess translates the two versions of the policy into formulas Pold and Pnew, respectively. It then searches for solutions to the formula (Pnew ∧ ¬Pold) that represent requests that are allowed by the new policy but not allowed by the old policy (“¬” is the mathematical symbol for “not”). Because the new policy allows principals in 123456789012 to perform an action that the old policy did not, the check fails, and a security engineer can review whether this policy change is acceptable.
With CheckAccessNotGranted, security engineers can be more prescriptive by specifying critical permissions to be checked against policy updates. Let’s say we want to ensure that developers are not granting permissions to delete an important bucket. In our previous example, CheckNoNewAccess detected this only because the permission was added with an update. With CheckAccessNotGranted, the security engineer can specify s3:DeleteBucket as a critical permission. We then translate the critical permissions into a formula such as (Action = “s3:DeleteBucket”) and search for requests with that action that are allowed by the policy. Because the preceding policy allows this action, the check fails and that prevents the permission from being deployed.
With the ability to specify critical permissions as parameters to the CheckAccessNotGranted API, you can now check policies against your standards — and not just for canned, broadly applicable checks.
Debugging failures
By democratizing policy checks, without the need for costly and time-consuming manual reviews, custom policy checks help developers move faster. When policies pass the checks, developers can make updates with confidence. If policies fail the checks, IAM Access Analyzer provides additional information so that developers can debug and fix them.
Suppose a developer writes the following identity-based policy:
{ "Version": "2012-10-17", "Statement": [ { "Effect": "Allow", "Action": [ "ec2:DescribeInstance*", "ec2:StartInstances", "ec2:StopInstances" ], "Resource": "arn:aws:ec2:*:*:instance/*" }, { "Effect": "Allow", "Action": [ "s3:GetObject*", "s3:PutObject", "s3:DeleteBucket" ], "Resource": "arn:aws:s3:::DOC-EXAMPLE-BUCKET/*" } ] }
Let’s also suppose that a security engineer has specified critical permissions that include s3:DeleteBucket. As described above, CheckAccessNotGranted fails on this policy.
For any given policy, it can sometimes be hard to understand why a check failed. To give developers more clarity, IAM Access Analyzer uses Zelkova to solve additional problems that pinpoint the failure to a specific statement in the policy. For the preceding policy, the check failed with the description "New access in the statement with index: 1". This description indicates that the second statement contains a critical permission.
The key to democratizing automated reasoning is to make it simple to use and easy to specify properties. With additional custom checks, we will continue to enable our customers on their journey to least privilege.